Humanising Machines | Por @OpenEXO
This essay is adapted from a transcript of the interview above. And was originally featured on Nell Watson's page here.
In the present age, Machine Learning is on the verge of transforming our lives. The need to provide intelligent machines with a moral compass is of great importance, especially at a time when humanity is more divided than ever. Machine Learning has endless possibilities, but if used improperly, it could have far-reaching and lasting negative effects.
Many of the ethical problems regarding Machine Learning have already arisen in analogous forms throughout history, and we will consider how, for example, past societies developed trust and better social relations through innovative solutions. History tells us that human beings tend not to foresee problems associated with their own development but, if we learn some lessons along the way, then we can take measures in the early stages of Machine Learning to minimize unintended and undesirable social consequences. It is possible to build incentives into Machine Learning that can help to improve trust through mediating various economic and social interactions. These new technologies may one day eliminate the requirement for state-guided monopolies of force and potentially create a fairer society. Machine Learning could signal a new revolution for humanity; one with heart and soul. If we can take full advantage of the power of technology to augment our ability to make good, moral decisions and comprehend the complex chain of effects on society and the world at large, then the potential benefits of prosocial technologies could be substantial.
THE EVOLUTION OF AUTONOMY
Artificial Intelligence (AI), which is also known as Machine Intelligence, is a blanket term that describes many different sub-disciplines. AI is any technology that attempts to replicate or simulate organic intelligence. The first type of AI in the 1950s and 1960s was essentially a hand-coded if/then statement (if condition “x,’ then do “y”). This code was difficult and time-intensive to program and made for very limited capabilities. Additionally, if the system encountered something new or unexpected, it would simply give an exception and crash.
Since the 1980s, Machine Learning has been considered a subset of AI whereby instead of programming machines explicitly, one introduces them to examples of what you want them to learn, i.e. ‘here are pictures of cats, and here are pictures of things like cats, but not cats, like foxes or small dogs.’ With time and through the use of many examples, Machine Learning Systems can educate themselves without needing to be explicitly taught. This is extremely helpful for two main reasons. First, hand coding is no longer necessary. Imagine trying to code a program to detect cats and not foxes. How do we tell a computer what a cat looks like? Breeds of cats can look quite different from one another. To do this by hand would be almost impossible. But with Machine Learning, we can outsource this process to the machine. Second, Machine Learning Systems have adaptability. If a new breed of cat is introduced, you simply update the machine with more data, and the system will easily learn to recognize the new breed without the need to reprogram the system.
More recently, starting around 2011, we have seen the development of Deep Learning Systems. These systems are a subset of Machine Learning and use many different layers to create more nuanced impressions that make them much more useful. It’s a bit like baking bread. You need salt, flour, water, butter, and yeast, but you can’t use a pound of each. They must be used in the correct proportion. These ingredients make simple bread if put together in the right amounts. However, with more variables, such as extra ingredients, and other ways to form the bread, you can make everything from a pain au chocolat to a biscotti. In a loose analogy, this is how Deep Learning compares to pure Machine Learning.
These technologies are very computationally intensive and require large amounts of data. But with the development of powerful graphics processing chips, this task has become much easier and can now be performed by something as small as a smartphone to bring machine intelligence to your pocket. Also, thanks to the internet, and the many people uploading millions of pictures and videos each week, we can create powerful sets of examples (datasets) for machines to learn from. The computing capacity and the data examples were critical prerequisites that have only recently been fulfilled and enabled this technology to finally be deployed, using algorithms invented back in the 1980s that were not usable at the time.
In the last two years, we have seen developments such as Deep Reinforcement Meta Learning, a subset of Deep Learning, where instead of learning as much as possible about one subject, a system tries to learn a little bit about a greater number of subjects. Meta-learning is contributing to the development of systems that can cope with very complex and changing variables and single systems that can navigate an environment, recognize objects, and have a conversation all at the same time.
THE BLIND HODGEPODGE MAKER
Despite the rapid advances in Machine Intelligence, as a society, we are not prepared for the ethical and moral consequences of these new technologies. Part of the problem is that it is immensely challenging to respond to technological developments, particularly because they are developing at such a rapid pace. Indeed, the speed of this development means that the impact of Machine Learning can be unexpected and hard to predict. For example, most experts in the AI space did not expect the abstract strategy game of Go to be a solvable problem by computers for at least another ten years. And there have been many other significant developments like this that have caught experts off guard. Furthermore, advancements in Machine Intelligence paint a misleading picture of human competence and control. In reality, researchers in the Machine Intelligence area do not fully understand what they are doing, and a lot of the progress is essentially based on ad hoc experimentation such that if an experiment appears to work, then it is immediately adopted.
To draw a historical comparison, humanity has reached the point where we are shifting from alchemy to chemistry. Alchemists would boil water to show how it was transformed into steam, but they could not explain why water changed to a gas or vapor, nor could they explain the white powdery earth left behind (the mineral residue from the water) after complete evaporation. In modern chemistry, humanity began to make sense of the phenomena through models, and we started to understand the scientific detail of cause and effect. We can observe a sort of transitional period where people invented models of how the world works on a chemical level. For instance, Phlogiston Theory was en vogue for nearly two decades, and it essentially tried to explain why things burn. This was before Joseph Priestley discovered oxygen. We have reached a similar point in Machine Learning as we have a few of our own Phlogiston type theories such as the Manifold Hypothesis. But we do not really know how these things work, or why. We are now beginning to create a good model and have an objective understanding of how these processes work. In practice that means we have seen examples of researchers attempting to use a sigmoid function and then, due to the promising initial results, trying to probe a few layers deeper.
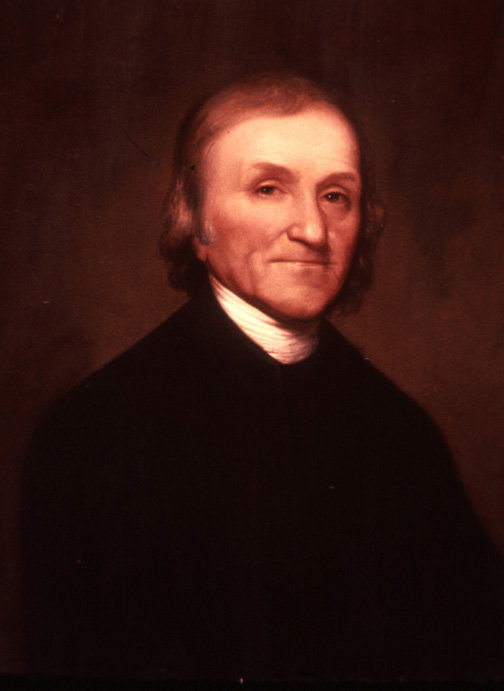
For instance, Phlogiston Theory was en vogue for nearly two decades and it essentially tried to explain why things burn. This was before Joseph Priestley discovered oxygen. We have reached a similar point in machine learning as we have a few of our own Phlogiston theories, such as the Manifold Hypothesis. For instance, Phlogiston Theory was en vogue for nearly two decades, and it essentially tried to explain why things burn. This was before Joseph Priestley discovered oxygen. We have reached a similar point in Machine Learning as we have a few of our own Phlogiston type theories such as the Manifold Hypothesis. But we do not really know how these things work, or why. We are now beginning to create a good model and have an objective understanding of how these processes work. In practice that means we have seen examples of researchers attempting to use a sigmoid function and then, due to the promising initial results, trying to probe a few layers deeper.
Through a process of experimentation, we have found that the application of big data can bring substantive and effective results, although, in truth, many of our discoveries have been entirely accidental with almost no foundational theory or hypotheses to guide them. This experimentation without method creates a sense of uncertainty and unpredictability, which means that we might soon make an advancement in this space that is more efficient by orders of magnitude more than we have ever seen before. Such a discovery could happen tomorrow, or it could take another twenty years.
DISSENT AND DIS-COHESION
In terms of the morality and ethics of machines, we face an immense challenge. Integrating these technologies into our society is a daunting task. These systems are little optimization genies; they can create all kinds of remarkable optimizations or impressively generated content. However, that means that society might be vulnerable to deceit or counterfeiting. Optimization should not be seen as a panacea. Humanity needs to think more carefully about the consequences of these technologies as we are already starting to witness the effects of AI on our society and culture. Machines are often optimized for engagement, and sometimes, the strongest form of engagement is to evoke outrage. If machines can get results by exploiting human weaknesses and provoking anger, then there is a risk that they may be produced for this very purpose.
Over the last ten years, we have seen a strong polarization of our culture across the globe. People are, more noticeably than ever before, falling into distinctive ideological camps which are increasingly entrenched and distant from each other. In the past, there was a strong consensus on the meaning of morality and what was right and wrong. Individuals may have disagreed on many issues, but there was a sense that human beings were able to find common ground and ways of reaching agreement on the fundamental issues. However, today, people are increasingly starting to think of the other camps, or the other ideologies, as being fundamentally bad people.
Consequently, we are beginning to disengage from each other, which is damaging the fabric of our society in a very profound and disconcerting way. We’re also starting to see our culture being damaged in other ways as seen in the substantial amount of negative content that is uploaded to YouTube every minute. It’s almost impossible to develop an army of human beings in numbers sufficient enough to moderate that kind of content. As a result, much of the content is processed and regulated by algorithms. Unfortunately, a lot of those algorithmic decisions aren’t very good ones, and so quite a bit of content which is, in fact, quite benign, ends up being flagged or demonetized for mysterious reasons that can’t be explained by anyone. Entire channels of content can be deleted overnight, on a whim and with little oversight or opportunity for redress. There is minimum human intervention or reasoning involved in trying to correct unjustified algorithmic decisions.
This problem is likely to become a serious one as more of these algorithms get used in our society every day and in different ways. This may be potentially dangerous because it can lead to detrimental outcomes and situations where people might be afraid to speak out. Not because fellow humans might misunderstand them, although this is also an increasingly prevalent factor in this ideologically entrenched world, but because the machines might. For instance, a poorly constructed algorithm might select a few words in a paragraph and come to the conclusion that the person is trolling another person, or creating fake news, or something similarly negative.
The ramifications of these weak decisions could be substantial. Individuals might be downvoted or shadowbanned with little justification, and find themselves isolated, effectively talking to an empty room. As the reach of the machines expands, there flawed algorithmic decision systems have the potential to cause widespread frustration in our society. It may even engender mass paranoia as individuals start to think that there is some kind of conspiracy working against them even though they may not be able to confirm why they have been excluded from given groups and organizations. In short, an absence of quality control and careful consideration of the complex moral and ethical issues at hand may undermine the great potential of Machine Learning and its impact on the greater good.
COORDINATION IS THE KEY TO COMPLEXITY
There are certainly some major challenges to be overcome with Machine Learning, but we now have the opportunity to make appropriate interventions before potentially significant problems arise. We still have an opportunity to change course to overcome them, but it is going to be a real challenge. Thirty years ago, the entire world reached a consensus on the need to cooperate on CFC (chlorofluorocarbon) regulation. Over a relatively short period of a few years, governments acknowledged the damage that we were inflicting on the ozone layer. They decided that action had to be taken and, through coordination, a complete ban on CFCs was introduced in 1996, which quickly made a difference in the environment. This remarkable achievement is a testament to the fact that when confronted with a global challenge, governments are capable of acting rapidly and decisively to find mutually acceptable solutions. The historical example of CFCs should, therefore, provide us with grounds for optimism in the hope that we might find cooperative ethical and moral approaches to Machine Learning through agreed best practices and acceptable behavior.
Society must move forward cautiously and with pragmatic optimism in engineering new technologies. Only by adopting an optimistic outlook can we reach into an imagined better future and find a means of pulling it back into the present. If we succumb to pessimism and dystopian visions, then we risk paralysis. This is similar to the type of panic humans experience when they find themselves in a bad situation. It is akin to drowning in quicksand slowly. In this sort of situation, panicking is likely to lead to a highly negative outcome. Therefore, it is important that we remain cautiously optimistic and rationally seek the best way to move forward. It is also vital that the wider public be aware of the challenges, but also aware of the many possibilities that exist. Indeed, while there are many dangers in relying so heavily on machines, we must recognize the equally significant opportunities they present to guide us and help us be better human beings, to have greater power and efficacy, and to find more fulfillment and greater meaning in life.
DATASET IS DESTINY
One of the reasons why Machine Intelligence has taken off in recent years is because we have extraordinarily rich datasets that are collections of experiences about the world that provide a source for machines to learn from. We now have enormous amounts of data, and thanks to the Internet, there is a readily available source offering new layers of information that machines can draw from. We have moved from a web of text and a few low-resolution pictures, video, and location and health data, etc. And all of this can be used to train machines and get them to understand how our world works, and why it works in the way it does.
A few years ago, there was a particularly important dataset that was released, by a professor called Fei-Fei Li and her team. This dataset, ImageNet, was a corpus of information about objects ranging from buses and cows to teddy bears and tables. Now machines could begin to recognize objects in the world. The data itself was extremely useful for training convolutional neural networks that were revolutionary new technologies for machine vision. But more than that, it was a benchmarking system because you could test one approach versus another, and you could test them in different situations. This capability led to the rapid growth of this technology in just a few years. It is now possible to achieve something similar when it comes to teaching machines about how to behave in socially acceptable ways. We can create a dataset of prosocial human behaviors to teach machines about kindness, congeniality, politeness, and manners. When we think of young children, often we do not teach them right and wrong, but rather we teach them to adhere to behavioral norms such as remaining quiet in polite company. We teach them simple social graces before we teach them right and wrong. In many ways, good manners are the mother of morality and essentially constitute a moral foundational layer.
THE RISE OF THE MACHINES
There is a broader area of study called value alignment, or AI alignment. It is centered around teaching machines how to understand human preferences and how humans tend to interact in mutually beneficial ways. In essence, AI alignment is about ensuring that machines are aligned with human goals. We do this by socializing machines so that they know how to behave according to societal norms. There are some promising technical approaches and algorithms that could be used to accomplish this, such as Inverse Reinforcement Learning. In this technique machines can observe how we interact and decipher the rules without being explicitly told; effectively by watching how other people function. To a large extent, human beings learn socialization in similar ways. In an unfamiliar culture, individuals will wait for other people to start doing something like how to greet someone or which fork to use when eating. Children learn this way, so there are many great opportunities for machines to learn about us in a similar fashion.
Armed with this knowledge, we can move forward by trying to teach machines about basic social rules; like it isn’t nice to stare at people; or to be quiet in a church or a museum; or if you see someone drop something that looks important, you should alert them. These are the types of simple societal rules that we might ideally teach a six-year-old child. If this is successful, then we can move on to more complex rules. The important thing to remember is that we have some information that we can use to begin to benchmark these different approaches. Otherwise, it may take another twenty years to teach machines about human society and how to behave in ways that we prefer.
While the number of ideas in the field of Machine Learning is a positive sign, we cannot realize them in practice until we have the right quality and quantity of data. My nonprofit organization, EthicsNet, is creating a dataset of prosocial behaviors which have been annotated or labeled by people of differing cultures and creeds across the globe. The idea is to gauge as wide a spectrum of human values and morals as possible and to try to make sense of them so that we can find the commonalities between different people. But we can also recreate the little nuances or behavioral specificities that might be more suitable to particular cultures or situations.
Acting in a prosocial manner requires learning the preferences of others. We need a mechanism to transfer those preferences to machines. Machine intelligence will simply amplify and return whatever data we give it. Our goal is to advance the field of machine ethics by seeding technology that makes it easy to teach machines about individual and cultural behavioral preferences.
There are very real dangers to our society if one ideologically-driven extremist group ever gains supremacy in establishing a master set of values for machines. This is why EthicsNet needs to continue its mission to enable a plurality of values to be collected and mapped and for a “passport of values” that will allow machines to meet our personal value preferences. Heaven help our civilization if machine values were ever to be monopolized by extremists. Many individuals and groups in the coming years will attempt to develop this as a deadly weapon. It is the ultimate cudgel to smash dissent and ‘wrongthink’. As a global community, we must absolutely resist any attempt to have values forced upon us via the medium of intelligent machines. This is a time of intense polarization and extremist positions when there is tremendous temptation to press for an advantage for one’s own tribe. Safeguarding a world where a plurality of values is respected requires the earnest efforts of people with noble, dispassionate, and sagacious characters.
No hay comentarios.